Transportation Networks and Technology
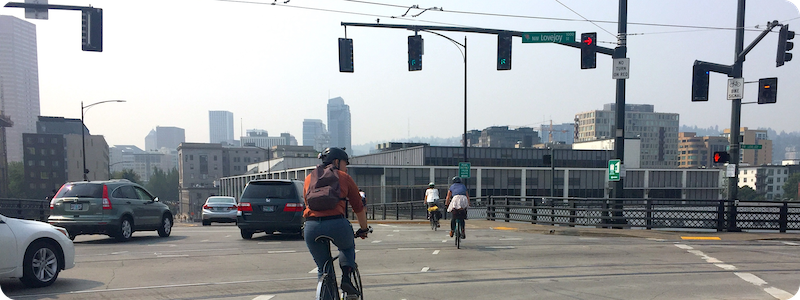
Travel time, operating speed and reliability all influence operating costs and system efficiencies, and in turn influences how people choose to get to their destinations. Researchers at PSU use the latest technology in optimizing models to achieve short-term and long-term positive impacts on our transportation networks in large-scale urban areas. Greater capacity for modeling and measuring the performance of our networks will lead to better infrastructure planning and management strategies, ultimately leading to reduced emissions and other related health benefits.
Learn more about some of our most impactful research on transportation networks and technology below, or see all our projects here.
NSF Collaborative Research: Non-Additive Network Routing and Assignment Models (Active Project)
Learn more about NSF Collaborative Research: Non-Additive Network Routing and Assignment Models (Active Project) led by Avinash Unnikrishnan.
Statistical Inference for Multimodal Travel Time Reliability (Active Project)
Travel time reliability is a key metric of interest to practitioners and researchers. Travel time reliability metrics have been defined for transit and automobiles, such as the ratio of percentile to sample mean; the ratio of percentiles to the median; etc. Traditionally, transportation engineers and planners have used point estimates to compare travel time reliability for various other modes. Ready-made procedures to perform statistical inference (i.e. confidence intervals and hypothesis tests) are not available for these metrics. This project will evaluate and develop methods to determine confidence intervals and hypothesis tests for select travel time reliability parameters. Another complicating factor is travel time distribution, which often varies depending on the location and time. Travel time distributions depend on facility type, traffic conditions, signal settings, and composition. The research team will focus on distribution-free confidence intervals and hypothesis test procedures which work for a wide range of shapes of distributions. The team will also study the applicability of existing travel time reliability metrics for class one vehicles (bicycles and motorbikes) and the feasibility of defining an overall travel time reliability of an arterial segment that considers all modes.
Learn more about Statistical Inference for Multimodal Travel Time Reliability (an active project), led by Avinash Unnikrishnan, Miguel Figliozzi, and Subhash Kochar.
Understanding Factors Affecting Arterial Reliability Performance Metrics
Learn more about Understanding Factors Affecting Arterial Reliability Performance Metrics, led by Avinash Unnikrishnan, Sirisha Kothuri and Jason Anderson.
A Decentralized Network Consensus Control Approach for Urban Traffic Signal Optimization
The research team created a graphical user interface (access on GitHub) that lets the user visualize the simulation runs in an easy-to-understand environment and provides direct access to a number of simulation parameters. Researchers also provided simple tools to analyze the simulation results offline. With the simulation tools created in this project, other researchers in traffic management can explore the potential of the network consensus protocol. The complete open source for the code is available in the online depository. This work could influence current practices regarding traffic light cycles, and could have an impact in alleviating congestion in urban streets.
Learn more about A Decentralized Network Consensus Control Approach for Urban Traffic Signal Optimization, led by Gerardo Lafferriere.
Using High-Resolution Bus Detection Data to Improve Travel Time Prediction and Identify Urban Congestion Spots
This research project explored potential applications of the new data for assessing transit performance, and for estimating transportation system performance measures for urban streets and arterials. A new inter-stop trip time model was developed using the five-second resolution data. This newly developed model resulted in statistically significant and improved results over previous models. The models for overall travel time indicated that dwell time and average speed between stops were the major factors influencing transit travel time. Estimation of average speed between stops is a critical component of the transit trip time models. The research provides conclusions that can be used by transit agencies to improve operations through improvements such as transit signal priority. More importantly, for transit agencies looking for ways to archive data the research provides recommendations on formatting the data that can be most useful for future analysis.
Learn more about Using High-Resolution Bus Detection Data to Improve Travel Time Prediction and Identify Urban Congestion Spots led by Miguel Andres Figliozzi.
The People
Meet the Portland State University experts behind many of the projects listed above:
- Jason Anderson, Research Associate, Civil and Environmental Engineering, Portland State University
- Miguel Figliozzi, Professor, Civil and Environmental Engineering, Portland State University
- Sirisha Kothuri, Senior Research Associate, Civil and Environmental Engineering, Portland State University
- Subhash Kochar, Professor, Mathematics and Statistics, Portland State University
- Gerardo Lafferriere, Professor, Mathematics and Statistics, Portland State University
- Avinash Unnikrishnan, Professor, Civil and Environmental Engineering, Portland State University